Over- And Under-Supplying Hurts Profits: A New Methodology To Help Retailers Improve Inventory Decisions
By Amr Farahat, professor of operations management, Washington University in St. Louis
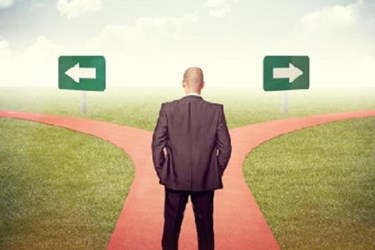
With the madness of Black Friday and holiday shopping behind us, retailers are taking stock of inventory. Matching expensive supply to uncertain demand each holiday season — and beyond — remains a central problem they and their suppliers face.
If retailers supply more product than demand, they are left with excess inventory which ties up capital and leads to obsolescence. Too little supply results in lost sales and weakens customer retention. Indeed, a study by retail analytics group DynamicAction found stock-outs, overstocks, and returns are costing retailers $1.75 trillion a year as supply chains become more complex and omni-channel retailing grows.
This central challenge facing retailers was the motivation for research I carried out with Joonkyum Lee, assistant professor at Sogang Business School in South Korea, into how retailers can improve inventory decisions. We focused on products whose replenishment lead-times are long compared to the duration of their peak selling season. We also focused on products whose customers exhibit significant stockout-based substitution and other inventory-dependent purchase behaviors commonly observed by retailers.
One common approach taken by retailers is to mitigate demand risk by signing contractual agreements with suppliers. Many college bookstores, for example, have a clause written in their contracts ensuring suppliers buy back books they cannot sell. This may help solve the issue of oversupply, but it does little to foresee demand. Retailers have to estimate expected customer traffic at different stores, which products customers may be looking for, and how they will behave if they don’t find the product they were initially looking for — product substitution.
Fortunately, advances in Big Data analytics and technology are transforming the process of demand forecasting. For example, the analysis of customer substitution patterns using state-of-the-art choice models is vastly improved and simplified when sales data is tracked in real-time. However, considerable uncertainty still remains in demand forecasts, especially at the SKU level.
So another approach is to be more responsive to demand by shortening lead times. Nike is a good example of a retailer doing this. Previously, the company’s product cycle began with an order made months in advance — 566 factories would then produce an estimated 1.3 billion units of goods to ship to 30,000 retailers in 190 countries with a lead time of 60 days. But Nike has shortened its lead time by 83.3 percent, to just 10 days, by using automation in its Asian suppliers’ factories. This means it can now “deliver what consumers want, when they want it, where they want it,” COO Erik Sprunk explains.
But this approach, too, has its limits. This is especially true during the holiday season when sales are concentrated into a few key dates and shoppers are not inclined to delay their purchases until replenishments arrive. Retailers need to anticipate customer demand and stock accordingly.
In our research, Professor Lee and I developed a data-driven methodology to tackle this problem of matching expensive supply to uncertain demand. It builds on the predictive analytics capabilities many large retailers are already developing by providing the prescriptive optimization piece. The methodology provides recommendations for retailers they can use as a starting point for their final inventory decisions.
Our algorithm, Approximate Similarity Transformation, examines mathematically the relationship between how much inventory retailers stock and their profits. This relationship is complicated because customer preferences, substitution patterns, and purchase journeys are so varied. We replace this relationship with a simpler one that slightly over-estimates expected profits and optimizes inventory levels based on this upper bound. Using this approach as a basis for inventory decisions yields better results than other approaches proposed in the research literature.
To demonstrate this, we constructed 66,825 problem instances across a range of products, profit margins, demand levels, and customer behavior patterns based on the Nested Logit and Generalized Attraction models. For each problem instance, we compared our method against two other leading benchmarks.
Our method yielded stocking solutions that met or exceeded the profit of the leading benchmarks most, though not all, of the time with 2 to 3 percent improvements in profit contribution being common. The gap between the profit proposed by our solution and the optimal profit was typically small. And the bounds generated by our method were more accurate than those of other benchmarks in 99 percent of the instances. We also found our method yielded faster computation times.
Given the significant profit potential and the relatively modest cost of investment in analytics capabilities, we hope retailers engage in testing and implementing this and other similar methodologies to better match supply and demand in future peak shopping seasons.
Resources
Our research paper, The Multi-Product Newsvendor Problem with Customer Choice, appears in Operations Research, a scholarly peer-reviewed journal. The Approximate Similarity Transformation code is publicly available at: https://github.com/joonkyumlee/Multiproduct-newsvendor/.
About The Author
Amr Farahat is a professor of operations management at Washington University in St. Louis. He recently served as visiting professor at MIT Sloan School of Management. Joonkyum Lee is a professor at Sogang Business School in South Korea.