Two Use Cases For Artificial Intelligence
By Matt Pillar, chief editor
What do modern customer engagement and inventory management applications have in common? Increasingly, they both leverage artificial intelligence.
I caught up with Omer Artun, CEO and founder of predictive analytics company AgilOne, in a crowded dining area at the Javits Center during last January’s NRF Show.
I found Doug Benson, director of America’s marketing at image recognition company Trax Image Recognition, about an hour later at the same event. Neither company has much in common, with the exception that both businesses are leveraging AI (artificial intelligence) to drive innovation to their retail customers.
On the one hand, you have a company that just released a multiple camera-wielding robot designed to troll store aisles gathering a comprehensive image of the contents of those stores’ shelves. That’s 6-year-old startup Trax Image Recognition, and its software is capable of recognizing multiple, nearly identical products, regardless of how funky the packaging or whether the view of the product is obstructed, with around 96 percent accuracy.
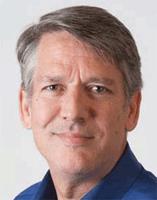
"Consumer product goods (CPG) manufacturers are big users because they spend so much time in stores visually analyzing shelves. ... Retailers want the same sort of image recognition functionality, but they want it across the entire store."
Doug Benson, director of America’s marketing, Trax Image Recognition
What’s the point of gathering these images? Benson says the applications are many. “Consumer product goods [CPG] manufacturers are big users because they spend so much time in stores visually analyzing shelves.” That analysis contributes to a bigger-picture understanding of what’s going right or wrong with the execution of marketing, promotions, merchandising, planogramming, and more. Combined with POS data, shelf presentation imagery can, for instance, help CPG merchandisers and marketers understand why a product might see sales success in one store while it struggles in another.
Shelf-Level Intelligence For Retailers
While the camera-armed robot is new, the theory behind Trax’s image recognition application is not. Benson says CPG manufacturers have been using the technology for the past five years to help manage specific categories, albeit via camera-wielding associates, not robots. The robot was developed not for manufacturers but for merchants concerned about on-shelf availability and its impact on sales, as well as expense reduction and the efficient capture of store-level information.
“Retailers want the same sort of image recognition functionality, but they want it across the entire store,” says Benson. While associates can certainly leverage the same manual image recognition application used by manufacturer representatives, the robot, built by San Jose-based Fetch Robotics and featuring three tiers of cameras, automates and accelerates the collection of images. By the first quarter of 2017, Trax was boasting more than a dozen pilot implementations. Benson says these pilots are showing some early proof that one best practice might entail the robots working an early night shift, automatically launching from their docking stations at a predetermined time, making their rounds, and parking themselves in their docking stations much like a Roomba does. Then, the images they’ve collected are uploaded to the Trax application in the cloud, where analysis occurs and from which stocking and merchandising directive reports are produced and delivered in advance of the night crew beginning its shift. Those reports, complete with store maps and multilevel shelf views of merchandise, inform the night crew as it prepares shelves for the following business day.
![]()
"There are certain tasks that humans can do, but we can’t do them as fast as computers. … Then, there are tasks that humans can do really well that computers struggle with."
Omer Artun, CEO and founder, AgilOne
There is some overlap between image recognition-based inventory, merchandise, and promotions management tools and more traditional product management tools like the ubiquitous bar code or RFID tags. All three technologies can tell you what’s on the shelf at any given time. Only images can show you what that shelf actually looks like.
“Artificial intelligence is a bullet point beneath all the processes that are run on the images,” says Benson. “It’s part of the process of identifying all the individual products in the picture and determining what’s there on the shelf, what’s not, and what needs to be done.”
In addition to driving daily data to store associates, the application also allows executives at headquarters to glean insight into the inventory performance of the entire store. With the results of its analysis available in the cloud, there are big benefits for CPG and retail executives alike. Directives are based on rules merchants themselves have set, and they might include planogram, price, promotional compliance, and share of shelf, to name a few. “Merchant executives receive scores based on their own rules,” says Benson. “This gives them a way to think visually about their stores and see the problem areas they face.”
Of course, store layouts change frequently and the robots must be kept abreast of those changes. Benson says an hour of training prepares store level associates to reprogram the robot’s path as needed. The Trax application is subscription-based, cost dependent on the size of the store and the volume of images processed. The robots will come at an additional, albeit undetermined, cost.
Algorithms Trump Assumptions
When Omer Artun and I finally navigated our way under the great glass ceiling at Javits to a concrete pier large enough to accommodate two people and a laptop, he gave me a glimpse into the gears that turn product development at AgilOne. Artun holds a Ph.D. in physics and computational neuroscience from Brown. One of his first jobs out of college was a consulting gig with McKinsey, which led to executive-level marketing positions with CDW and Best Buy. In 2006 he founded AgilOne, intent on exploring every possible avenue of applying machine learning to retail marketing.
Artun’s premise is simple. “There are certain tasks that humans can do, but we can’t do them as fast as computers. Structured tax calculation programs come to mind,” says Artun. “Then, there are tasks that humans can do really well that computers struggle with, such as processing cues from ‘noisy’ environments to draw conclusions.” It’s his intent to develop the machine’s ability to more closely mimic how humans learn and retain information and apply that ability to marketing.
“Retail marketing is the perfect use case for artificial intelligence,” says Artun. “No two humans exhibit the exact same shopping habits, and picking up on those habits is challenging in a noisy environment like retail where shopper data sets are typically incomplete.”
Doug Benson, director of America’s marketing, Trax Image Recognition
Humans have a set of heuristics, defined as that which enables a person to discover or learn something for themselves. In computer science, a heuristic is loosely defined as an approach to problem solving — or in this case, artificial learning — that’s not guaranteed to be optimal or perfect, but sufficient for practical intentions. So, while it may be impossible for a computer to predict what will trigger a buying response from every single one of a million customers, artificial intelligence makes it entirely possible for that computer to determine what will increase the odds of a purchase among a segment of those customers who share common attributes. The more granularly those segments are articulated, the more valuable the sales and promotion impact.
While the fact-based establishment of dozens, hundreds, or even thousands of these buyer persona segments would be arduous work for humans, it’s merely an algorithmic exercise for computers. Machine learning makes it possible for computers to gain artificial intelligence by using structured calculations to chart what’s anticipated versus what actually happens, then draw a conclusion about that scenario moving forward. That, says Artun, lends itself to retail marketing, where the “what’s anticipated” is what you might think, hope, or attempt, while the “what actually happens” is the consumer’s end behavior (i.e., purchase, no purchase, abandoned cart, etc.).
Applied to marketing, artificial intelligence can make customer-specific determinations, such as whether a customer is likely to buy or engage if they’re sent a promotional email, how that likelihood is affected if the email contains a discount, or what a specific customer’s lifetime value might be. “The ability to make predictions about customers and drive them to certain actions is a powerful and valuable thing,” says Artun. “If, for instance, you know who needs a discount to buy and who doesn’t, you can get surgical about your offers and maximize your revenue.”
Omer Artun, CEO and founder, AgilOne
Artun explains that this “likely to buy” scenario is an example of supervised learning where the machine is trying to predict an outcome. Equally important to the success of artificial intelligence in retail, he says, is unsupervised learning, which is a bit more arbitrary. By “listening” to what people say and “observing” what they do on e-commerce and social media sites, machines can learn from the reinforcement of consumers’ actions. “The more reinforcement we can gather, the more likely we’ll be able to accurately and proactively recommend products shoppers are likely to purchase, or offer next-best alternatives for products that might be out of stock,” says Artun.
Who Needs Artificial Intelligence?
Which retailers is AI right for? While it’s somewhat obvious that the aforementioned image recognition/artificial intelligence technology duo promises particular benefits to the likes of grocers and convenience stores, customer segmentation enabled by machine learning has broad appeal to any retailer that sees frequent and fast-paced SKU changes, price changes, and inventory turn. “Anywhere you need to more clearly understand the relationship between the customer and the product and apply analytics at the point of execution makes artificial intelligence a good fit,” says Artun. The question, then, becomes not who needs it, but who doesn’t?